My article in Data Driven Investor published 10th of January2020 on AI as an Efficient Banker.
The most useful innovations that have emerged in today’s financial industry would not be possible without some basic capabilities of artificial intelligence. The main ones are to process large volumes of data, perform predictive operations and conduct real-time analyses of information sets. These alter not only the way banks and insurance companies operate but also the way customers behave. According to Bain & Company, the savings made possible by the deployment of AI will run up to $1.1 trillion by 2030, amounting to a 22 percent reduction in operating expenses. These figures are consistent with the assessments of Accenture.
What follows is an overview of how smart technologies are changing the face of finance and an attempt to predict such changes going forward. What can we expect AI to do for the financial and insurance industries?
Uniform tools to feed data to AI
Apart from money, banks’ most valuable asset is knowing their customer. For years, customers have been researched in traditional ways and targeted with standard marketing. This approach has had one fundamental flaw. Customer data was gathered and processed using mutually incompatible techniques and tools. Marketing, customer service and sales each relied on a different data collection technique. The digital revolution made it possible to consolidate and harmonize these disparate areas. Today, customer ratings, research on customer preferences and potential, product development and sales all take place in a shared digital space using mutually integrated and mutually compatible tools. Data, the “lifeblood” of AI, maybe fed from different sources but its underlying digital nature is a constant.
Real time instead of history
Importantly, the majority of these processes take place in real time. For a long time, the most essential variables for building solid customer relationships were age, income, occupation, marital status and history of relationships with relevant financial institutions. Banks still continue to use all of them. But they form only a part of the analytical puzzle. There are a number of new factors these days, which include observing the current activities of customers (online activities, of course). Companies learn what customers want and who they are by analyzing their behaviors on bank websites, in hotline conversations and in email and telephone interactions. Adding further to the significance of this information is the ability to process it in ways that are virtually unlimited, and, most importantly the fact it can be processed in real time.
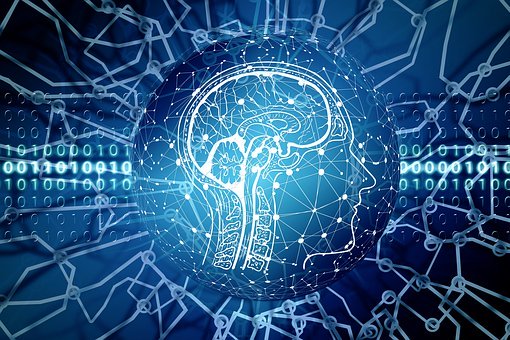
A voice assistant inquires about a loan
One of the most dramatic changes to be expected in the coming years will affect customer service. The sector will see a rise in the use of voice bots – the kind we are already familiar with our personal lives. Some go as far as to call this an upcoming “Alexization” of our lives. The term is a clear reference to the growing popularity of devices that support voice assistants, such as Alexa and Siri. The RBC Capital investment fund predicts that close to 130 million devices directly connected to Alexa will operate globally by 2020.
There is no reason why software based on the concepts utilized by such programs shouldn’t serve bank customers. All the more so given that bots trained by experts are increasingly better at imitating human interactions. Bank of America has deployed the chatbot Eric to advise bank customers with voice and text messages. The bot works 24 hours a day supporting all regular transactions that customers normally perform. This saves the bank bundles by replacing over a dozen if not dozens of specialists doing shift work 24/7. In Silicon Valley, they say that unlike machine algorithms and the chatbots they support, humans are not scalable.
When a bot sounds like a human
Since self-learning machines improve over time, assistant devices will become increasingly more competent. A bot capable of carrying a conversation will no longer limit itself to answering questions about the weather or traffic. It may just as well advise you on loan interests and the benefits of opening a deposit. It can tell you how much money you need to repay and remind you about an upcoming payment. Today, devices of this sort do well in first contact with customers, sorting hotline callers, and putting customers through to appropriate departments. In the future, the duties of assistants will become more complex. Obviously, for this to happen, the bots’ communication skills will require some honing. Skills such as using compound sentences, tuning into customers’ intonation, and sensing their underlying problems are still in development. But the pace at which bots are learning is ever faster.
Money with a fingerprint
As a sector that collects and processes enormous data sets, banking faces a serious challenge. What makes it all the more serious is the fact that today’s users are highly sensitive to security threats. Their anxieties are compounded by regular media reports on leaks of sensitive data kept by companies and portals. Banking and insurance IT experts have their hands full searching ceaselessly for ways to restore the recently undermined confidence of the average consumer.
Against this background, it is interesting to note the gradual changes in the way accounts are accessed online. In a nutshell, this concerns logging and authentication procedures. The traditional approach relies on nothing other than the usual logins based on a string of characters. As hacking techniques improve, this becomes a critical vulnerability of information systems. Bots designed specially to steal such data intercept security codes and passwords. This calls for alternative solutions. By all indications, authentication may be revolutionized by the use of fingerprints and, coming up soon, face recognition. This unique, personal identifier appears to offer the best and also the easiest-to-use protection against system intrusions available today. Just as voice-enabled devices can made traditional search engines and manual data entry interfaces obsolete, character-string-based authentication will be rendered outdated by systems relying on the touch of the human hand.
Modern banker. Send us a selfie and we will give you a loan
And what about authentication based on a facial photograph? Is it even possible? It is beginning to be. The individual features preserved in a photograph represent unique content that cannot be forged. This uniqueness can be uncoded by thorough analysis using software that identifies even the smallest nuances of an image. The use of a scanner to enter a photo or the use of a laptop camera to scan in a face may well become the next authentication method. AI’s ability to recognize faces, which is increasingly valued, not least by the police, can be used in all kinds of contexts. One can imagine loans being granted on the basis of signals “written” on a photo, which we’ll upload into a designated space. Algorithms will be able to examine an image to provide a bank with information about our health and overall life situation, allowing the bank to assess our value.
Investigations and real-time surveillance
In view of these security considerations, it is also worth mentioning the use of AI tools to monitor the security of banking systems. Intelligent AI-based software is designed to track the smallest anomalies indicative of a hacker attack in real time. Every day, hundreds of thousands of hacks and theft attempts targeted at valuable data or financial assets occur world-wide. Old type software would be unable to detect such attacks due to their sheer number. It takes AI tools to provide adequate protection and a sense of security.
And it is not just about such attacks. Banks struggle with the constant natural challenge of having to process and approve ambiguous high-risk transactions in cases that allow for multiple interpretations. The difficulties arise in a wide range of fields from lending to loan repayments, to interest rate calculations, to a host of accounting operations. People using traditional devices need hours to analyze cases and reach decisions to either approve or reject operations. Systems that rely on machine learning can authorize such transactions within mere seconds. The benefits to system efficiency and security are plain to see.
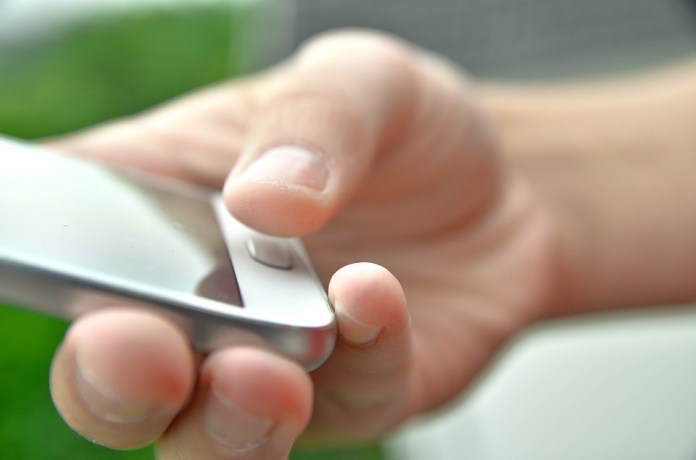
AI goes into insurance
These solutions are currently in use or will be used also in the insurance business. Here, too, artificial intelligence will be confronted with substantial amounts of data on customer behaviors and needs. This industry’s preoccupation with risk is even greater than that of banking. It employs algorithms to assess threats to customers’ life or health and examine their property holdings (real estate). It relies on the above-mentioned photo analysis technique. The sale of life insurance policies will require extensive analysis. From this viewpoint, new ways of gathering data attract much interest.
One possible source of massive amounts of information is the user’s car. Data on vehicle mileage, the driver’s accident proneness, and even driving style can be invaluable for companies developing personalized insurance products. It may also be vital to use advanced algorithms to assess the likelihood of customers developing health conditions by living specific lifestyles. We are yet to see whether future insurers will want to analyze bottom coded values, but the possibility cannot be ruled out.
Services tailored to customers and automated buying
AI will soon be able to perform the crucial task of reliably identifying people. This very ability will allow companies to handle every customer as a whole separate case rather than lumping like persons into larger sets. At a more general level, this innovation will enable providers to tailor their products and services to the needs of individual customers. There is also another solution that this approach will support. In the near future, customers will use interfaces that themselves analyze the data they enter. This step in automating banking services as a logical consequence of deploying smart algorithms.
Shopping for Mr. Smith and traders
Continuing along this train of thought, it is worth noting that automation will increasingly support product purchases. While today’s sales personnel continues to be an indispensable part of customer relationships, future customers will make do with mere applications. These may, for example, conduct serial sales of financial and insurance products and relieve customers of time-consuming decisions. Note that serial buying will be a life-saver for business investors who handle large volumes of products and information daily. Professional traders will be able to count on AI to relieve them of many duties, which today are associated with both shopping and extensive analyses.
The power of algorithms and the mystery of the black box
Artificial intelligence can count on a very secure future in banking and insurance. Algorithms are poised to simplify many operations for the convenience and time savings of customers. The sense of security that automation will give customers will become another revenue driver. As a side effect, one can undoubtedly expect impacts on employment in the financial sector. One case in point is the bank JP Morgan, which has launched an application (a platform) to extract data from loan applications. It would take the bank’s employees 360,000 hours to go through 12,000 such documents. Machine-learning software completes the job in, wait for it, a few hours. The benefits are evident. It is interesting to consider the event in terms of its impacts on employment policies.
Another vital question concerns explaining the inner workings of such algorithmic operations at a deeper level for both the sense of security of the customer and to show banks they are in a position to know what their system is doing. This brings us to the recently topical issue referred to as the “black box” problem. It concerns everything that happens inside AI-run devices and that often defies human understanding.
Similarly to a lense, the revolution sweeping through financial markets brings into focus all the key issues associated with the presence of AI in our personal and business lives. The only possible answer to the question regarding AI’s potential and its impact on the convenience of customers (financial product consumers) is: both the potential and the impacts are huge and unlike anything, we have seen before. The financial sector is one of the largest beneficiaries of algorithms’ rise to dominance in many industries. Such industries are nevertheless acutely aware of the scores of unanswered questions on the protection of funds, system security, customer data processing and regulation. One needs AI to be able to use the money to make more money. But money also requires rules and regulations so that it doesn’t evaporate rapidly amidst technological turmoil.
Link to this article
Related articles
– Technology 2020. Algorithms in the cloud, food from printers and microscopes in our bodies
– Learn like a machine, if not harder
– Time we talked to our machines
– Will algorithms commit war crimes?
– Machine, when will you learn to make love to me?
– Hello. Are you still a human?
TomK
I see crypto everywhere
Guang Go Jin Huan
Right. That’s the kind of interactions we want for our robots. We want to mimic the human-human interaction
TomK
Current accounts have long been an entry point for banks, viewed as a way to build loyalty to sell products. With the likes of Google launching their accounts services, we’re now entering the era of the bank account as a commodity. For these new entrants, offering accounts or ways to store and pay money is less about payments, and much more about ensuring that their platforms offer additional benefits to customers in terms of convenience, product range or personalised approach.
Acula
Well despite progress in AI it is still quite stupid. And we hard time make it more intelligent that say, a worm. This is because expanding resources available to AI (a trick that worked for regular computers) tends to make AI more stupid rather than more intelligent as having such capability it tends to memorize instead of generalizing. Hence creating complicated AI systems capable of thought seems to be well ahead of us.
The future is just an illusion in physics. This is just one possible way of ordering events. And not particularly remarkable one – outside of the way ur mind operates – that we can remember the past but cannot remember the future.
Mac McFisher
man I hope this happens. literally the only thing that gives me hope.
Adam T
Good read. This is future
Pico Pico
Blockchain and Fintech rulez 🙂
Mac McFisher
Personally, I think we’re already there, it just isn’t cost effective yet. And we’d have to do it the hard way. Based on experiments with segments of rodent brains, we could simulate a full human brain now if we had dump trucks of money to throw at it. The procedural/programmatic (not simulating a full brain) approach probably won’t happen until after someone does that, and that’s not going to happen until the hardware to do it is cheaper. Which, IMO, makes 90 years an optimistic estimate. As much as I make fun of the 10-15 year thing, I think it’s true if you assume unlimited funding.
JackC
Technology is slowing down but fortunately not computers and so many non Americans like me can fortunately witness big events in real time and also listen to potential heroes. Great job u/ultramarathonman . Hope you get peter thiel or patrick collison sooner. Both have a great take on the stagnation of technology and as someone in CS, maybe you will be forced to expand your pool of knowledge a bit more.
Zoeba Jones
https://hbr.org/podcast/2020/10/how-gpt-3-is-shaping-our-ai-future
AKieszko
As someone who specializes in artificial intelligence, I can say you don’t really understand it either.
AI is, at its very core, a filter between input and output. It can be anything, from linear regression to decision trees (which are basically a series of if/else). The only extra to it is that the model is able to “learn”, which is just optimizing functions from datasets, or a heuristics approach.
What most people seem to forget, is that neural networks are just big, refined decision trees. A long series of yes/no from input to decision. Our brain is litterally just a complex series of if/else. Although the plasticity and its vastness and feedback loops allows us to grasp extremely complex concepts.
After all, what does it mean for a human to be intelligent ? As I recall, atoms and laws are in motion, and that’s all, choices are not really choices, and in that context, are we that different from artificial intelligence ? Biology had millions of years to optimize our brain, technology just needs to catch up.
John Accural
Data always has to be shared. Having a baby is a data producing event that requires the sharing of that data between hospital, parents, and government. Baby gets a number for their own data file that will be added to until after they die.
No matter who owns the data (a shared ownership) no one actually owns the baby. The baby will have its own rights to its copy of the data but others also have the right to copy that data for verified purposes.
Simon GEE
This is actually quite alarming that a company/industry that does everything possible to avoid regulation of products they have financial interests in is calling for regulation.
Jacek B2
Great read Norbert
Oniwaban
Interesting post. “Since self-learning machines improve over time, assistant devices will become increasingly more competent.” Do you think that this is a matter of 2-3 years or we need to wait like 5-10 years for bots’ improvement? I used bots in a financial industry (as a customer) several times and it always ended with a need of human support.
Mac McFisher
Most “AI” work today has dropped the idea of general intelligence and instead is focused on task intelligence i.e. getting good at doing 1 well defined goal. Especially with our current methods of developing AI, you’d have to gather a giant dataset for each possible task (which may be infinite). Not hard to see how thats currently intractable. General AI won’t come about (imo) until we have made serious serious progressions in the field of neuroscience, because the only example of general intelligence we have, we still know very little about
John Accural
And if bots discriminate based on race when they’re built to predict criminal factors, why should we be ignoring intelligence?
Only a fool ignores a valid source of data
Kinda like how, when you build systems that ignore race and gender, it’s white and asian men that most get picked for logical and heavily mental labor roles, and women for emotional labor roles, not because of their skin color and gender, but rather because their skin color and gender correlate to the skills required for success in the role, and the AI learns that, independent of the political bullshit.
TomK
The use of cash was already dropping before the pandemic, with one study from Access to Cash predicting the UK could see less than one in 10 payments involve physical money by the end of the 2020s. With concerns that physical cash could be a way of transmitting the virus, the use of coins and notes has dropped significantly, and it is unlikely to switch back once the pandemic has passed as consumers opt for contactless alternatives.